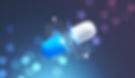
For many of those who want to understand how drug discovery can take place using Artificial Intelligence, this article would give a deeper understanding of the existing Neural Networks / Machine learning models which can be put to use to solve the long-prevailing problem of huge money and time investment. Even after so much effort, the drug discovered has 92% of chances to fail. Interested Artificial Intelligence Experts can go through the article to understand how this can be done. I will release a detailed artificial after this which will cover the NN Models mentioned below in detail. You can follow the list of articles here: zeeshaanpathan.com
Using Variational Autoencoders to Design Drugs:
Variational autoencoders (VAEs) defined over SMILES string and graph-based representations of molecules promise to improve the optimization of molecular properties, thereby revolutionizing the pharmaceuticals and materials industries. However, these VAEs are hindered by the non-unique nature of SMILES strings and the computational cost of graph convolutions. To efficiently pass messages along all paths through the molecular graph, researchers encode multiple SMILES strings of a single molecule using a set of stacked recurrent neural networks, pooling hidden representations of each atom between SMILES representations, and use attentional pooling to build a final fixed-length latent representation. By then decoding to a disjoint set of SMILES strings of the molecule, Researchers' All SMILES VAE learns an almost bijective mapping between molecules and latent representations near the high-probability-mass subspace of the prior. Researchers' SMILES-derived but molecule-based latent representations significantly surpass the state-of-the-art in a variety of fully- and semi-supervised property regression and molecular property optimization tasks. (Alperstein, Cherkasov and Rolfe, 2019) 2. Using TrimNet to outperform various previous AI models for Drug- Discovery: Computational methods accelerate drug discovery and play an important role in biomedicine, such as molecular property prediction and compound–protein interaction (CPI) identification. A key challenge is to learn useful molecular representation. In the early years, molecular properties are mainly calculated by quantum mechanics or predicted by traditional machine learning methods, which requires expert knowledge and is often labor-intensive. Nowadays, graph neural networks have received significant attention because of the powerful ability to learn representation from graph data. Nevertheless, current graph-based methods have some limitations that need to be addressed, such as large-scale parameters and insufficient bond information extraction. Results: In this study, researchers proposed a graph-based approach and employed a novel triplet message mechanism to learn molecular representation efficiently, named triplet message networks (TrimNet). Researchers show that TrimNet can accurately complete multiple molecular representation learning tasks with significant parameter reduction, including the quantum properties, bioactivity, physiology, and CPI prediction. In the experiments, TrimNet outperforms the previous state-of-the-art method by a significant margin on various datasets. Besides the few parameters and high prediction accuracy, TrimNet could focus on the atoms essential to the target properties, providing a clear interpretation of the prediction tasks. These advantages have established TrimNet as a powerful and useful computational tool in solving the challenging problem of molecular representation learning. (Li et al., 2020). 3. Use of Junction Tree variational autoencoder: In this model, researchers seek to automate the design of molecules based on specific chemical properties. In computational terms, this task involves continuous embedding and generation of molecular graphs. Researchers' primary contribution is the direct realization of molecular graphs, a task previously approached by generating linear SMILES strings instead of graphs. This junction tree variational autoencoder generates molecular graphs in two phases, by first generating a tree-structured scaffold over chemical substructures, and then combining them into a molecule with a graph message-passing network. This approach allows researchers to incrementally expand molecules while maintaining chemical validity at every step. Researchers evaluate the model on multiple tasks ranging from molecular generation to optimization. Across these tasks, the model outperforms previous state-of-the-art baselines by a significant margin. (Jin, Barzilay and Jaakkola, 2021) 4. Using Transferable Multi-level Attention Neural Network for Accurate Prediction of Quantum Chemistry Properties via Multi-task Learning. The development of efficient models for predicting specific properties through machine learning is of great importance for the innovation of chemistry and material science. However, predicting electronic structure properties like frontier molecular orbital HOMO and LUMO energy levels and their HOMO-LUMO gaps from the small-sized molecule data to larger molecules remains a challenge. Here researchers develop a multi-level attention strategy that enables chemical interpretable insights to be fused into multi-task learning of up to 110,000 records of data in QM9 for random split evaluation. The good transferability for predicting larger molecules outside the training set is demonstrated in both QM9 and Alchemy datasets. The efficient and accurate prediction of 12 properties including dipole moment, HOMO, and Gibbs free energy within chemical accuracy is achieved by using this specifically designed interpretable multi-level attention neural network, named as DeepMoleNet. Remarkably, the present multi-task deep learning model adopts the atom-centered symmetry functions (ACSFs) descriptor as one of the prediction targets, rather than using ACSFs as input in a conventional way. The proposed multi-level attention neural network applies to high-throughput screening of numerous chemical species to accelerate rational designs of a drug, material, and chemical reactions. (Liu et al., 2020). 5. Using MXMNet for predicting the physicochemical properties from molecular structure. The prediction of physicochemical properties from molecular structures is a crucial task for artificial intelligence-aided molecular design. A growing number of Graph Neural Networks (GNNs) have been proposed to address this challenge. These models improve their expressive power by incorporating auxiliary information in molecules while inevitably increase their computational complexity. In this work, researchers aim to design a GNN which is both powerful and efficient for molecule structures. To achieve such a goal, they propose a molecular mechanics-driven approach by first representing each molecule as a two-layer multiplex graph, where one layer contains only local connections that mainly capture the covalent interactions and another layer contains global connections that can simulate non-covalent interactions. Then for each layer, a corresponding message passing module is proposed to balance the trade-off of expression power and computational complexity. Based on these two modules, researchers build Multiplex Molecular Graph Neural Network (MXMNet). When validated by the QM9 dataset for small molecules and the PDBBind dataset for large protein-ligand complexes, MXMNet achieves superior results to the existing state-of-the-art models under restricted resources. (Zhang, Liu, and Xie, 2020) References: Alperstein, Z., Cherkasov, A. and Rolfe, J. T. (2019) All SMILES variational autoencoder, arXiv. Jin, W., Barzilay, R. and Jaakkola, T. (2021) Chapter 11: Junction Tree Variational Autoencoder for Molecular Graph Generation, RSC Drug Discovery Series. DOI: 10.1039/9781788016841-00228. Li, P. et al. (2020) 'TrimNet: learning molecular representation from triplet messages for biomedicine', Briefings in Bioinformatics, 00(September), pp. 1–10. DOI: 10.1093/bib/bbaa266. Liu, Z. et al. (2020) Transferable Multi-level Attention Neural Network for Accurate Prediction of Quantum Chemistry Properties via Multi-task Learning. DOI: 10.26434/chemrxiv.12588170. Zhang, S., Liu, Y. and Xie, L. (2020) Molecular mechanics-driven graph neural network with the multiplex graph for molecular structures, arXiv.